Predictive analytics is revolutionizing data management by leveraging innovative algorithms and a variety of data sources to transform how data is stored, accessed, and utilized. This evolution enhances the efficiency and security of data management, making predictive analytics essential for crafting smarter decision-making frameworks and enhancing business processes. By anticipating future trends and outcomes, businesses can craft proactive strategies, ensuring a competitive advantage. Additionally, predictive analytics improves operational strategies by identifying patterns and trends, optimizing resource allocation, and boosting customer satisfaction. As we explore further, we uncover how predictive analytics not only guides decision-makers towards best outcomes but also identifies growth opportunities, enhancing overall business insight.
Key Takeaways
- Predictive analytics enhances real-time data access and insights for dynamic decision-making.
- AI-driven threat detection evolves data security, safeguarding against sophisticated cyber threats.
- Cloud-based storage systems offer dynamic and scalable solutions, transforming how data is secured and accessed.
- Regulatory compliance is streamlined through predictive analytics, ensuring data management aligns with evolving laws.
- Automation in data governance reduces human error, improving accuracy and reliability in data handling.
The Predictive Analytics Landscape
The predictive analytics landscape is rapidly evolving, characterized by innovative algorithms and an increasing variety of data sources that are transforming how organizations approach data management. As this field advances, it is essential that we navigate the terrain with a keen awareness of ethical considerations, especially regarding algorithm bias. This bias, often unintentional, can skew outcomes and decisions, impacting fairness and objectivity.
For organizations desiring control over their predictive analytics strategies, it is vital to implement practices that identify and mitigate these biases. This involves rigorous testing of algorithms for fairness and accuracy, ensuring that decisions made by predictive models are both ethical and reliable. Transparency in how data is collected, processed, and applied within these models is essential. By acknowledging and addressing these concerns, organizations can harness the power of predictive analytics while maintaining ethical standards and trust.
Data Management Evolution
Understanding the ethical implications of predictive analytics paves the way for discussing the subsequent phase in data management's evolution. The shift towards data-centric architectures necessitates an exploration of advanced storage solutions and an unwavering commitment to regulatory compliance. These elements are not merely operational necessities but the foundation upon which predictive analytics stands, offering a control mechanism to navigate the complexities of modern data management.
Aspect | Evolution |
---|---|
Storage Solutions | From static repositories to dynamic, cloud-based systems. |
Data Accessibility | Enhanced with AI to provide real-time, actionable insights. |
Regulatory Compliance | Stricter adherence, with predictive analytics ensuring alignment. |
Data Security | Evolving through AI-driven threat detection and response mechanisms. |
This evolution underscores a transformation not just in how data is stored, but also in how it is secured, accessed, and utilized, ensuring that entities remain compliant and ahead in the competitive landscape. The progression towards more sophisticated storage solutions and the integration of compliance measures into the fabric of data management frameworks underscore a strategic pivot towards sustainability, security, and efficiency in the era of Big Data.
Smarter Decision-Making Frameworks
In today's data-driven landscape, smarter decision-making frameworks are emerging as essential tools for harnessing the power of predictive analytics to inform strategic choices. These frameworks integrate behavioral insights and thorough risk assessment methodologies, enabling organizations to navigate the complexities of modern business environments with greater precision. By leveraging predictive analytics, companies can anticipate future trends and outcomes, thus crafting strategies that are not only reactive but also proactive.
The incorporation of behavioral insights into these frameworks allows for a more nuanced understanding of customer and employee behaviors, which in turn informs more effective decision-making. Similarly, thorough risk assessment capabilities ensure that potential challenges and obstacles are identified and mitigated in advance, empowering leaders with the confidence to make bold decisions.
Ultimately, smarter decision-making frameworks represent a significant evolution in how data is utilized to guide strategic choices. By providing a structured approach to interpreting and acting on predictive analytics, these frameworks offer a clear path to achieving competitive advantage and operational efficiency. Therefore, they are indispensable for any organization seeking to maintain control in an increasingly uncertain and rapidly changing business landscape.
Enhancing Business Processes
Predictive analytics is revolutionizing the enhancement of business processes by enabling more efficient and effective operational strategies. By leveraging this powerful tool, companies are now able to anticipate customer needs, streamline operations, and greatly boost customer satisfaction. The implications for operational efficiency are profound, offering a competitive edge that is hard to rival.
Incorporating predictive analytics into business processes allows for the identification of patterns and trends within vast amounts of data, enabling organizations to make informed decisions that directly impact customer satisfaction. This proactive approach to management not only improves the customer experience but also optimizes resource allocation, ensuring that efforts are concentrated where they are most effective.
Furthermore, predictive analytics facilitates the fine-tuning of operational processes, identifying inefficiencies and potential areas for improvement. This leads to a more streamlined operation, minimizing waste and maximizing productivity. The end result is a significant enhancement in operational efficiency, directly contributing to the bottom line.
Predictive Models and Data Accuracy
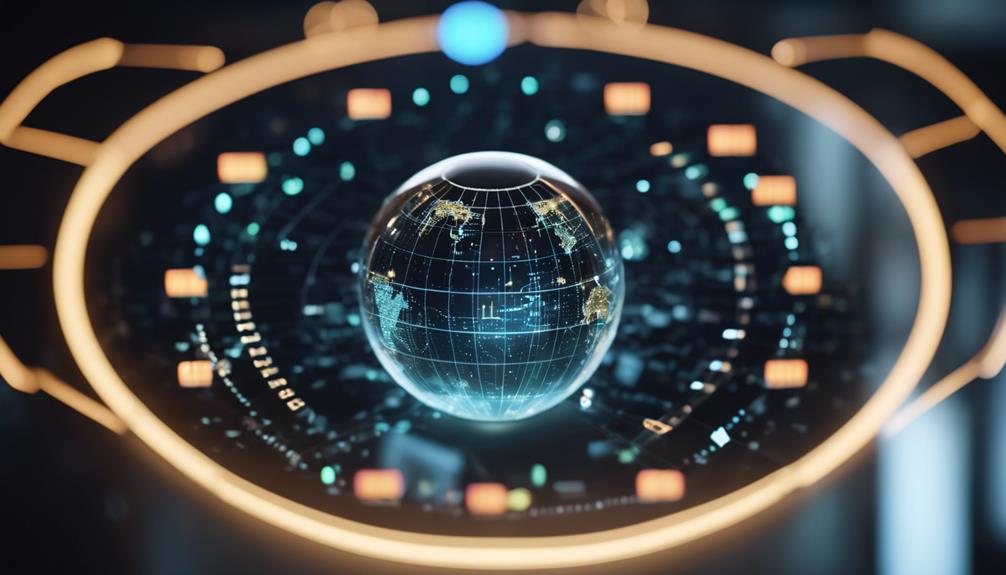
The accuracy of predictive models is foundational to the successful application of predictive analytics in data management and decision-making processes. Guaranteeing that these models generate reliable predictions demands a keen focus on data accuracy, which hinges on several critical factors:
- Model validation: Rigorous testing against unseen data sets to verify model reliability.
- Error analysis: Systematic examination of prediction errors to refine models.
- Quality of input data: Clean, relevant, and representative data sets lead to more accurate predictions.
- Algorithm selection: Choosing the right predictive modeling technique for the task at hand.
- Continuous updates: Models must evolve with new data to maintain their accuracy over time.
Professionals aiming for control in their strategic decisions understand that these elements are not just technical checkpoints but the backbone of a predictive analytics strategy that delivers results.
Model validation and error analysis, in particular, serve as the critical assessment stages where theoretical accuracy is put to the test in practical scenarios. This rigorous approach to validating predictive models ensures that decision-makers can rely on the insights provided, making data-driven strategies not just an option but a necessity for maintaining competitive advantage in today's fast-paced business environment.
AI Automation in Predictive Analytics
As the landscape of data management continues to evolve, AI automation emerges as a pivotal tool in predictive analytics. It promises to redefine how we approach streamlining data processing, enhancing accuracy levels, and forecasting future trends.
This technological advancement not only accelerates the speed at which data is analyzed but also greatly improves the reliability of the insights generated, setting a new standard for decision-making processes. By harnessing the power of AI automation, businesses are positioned to not only predict future trends with greater precision but also to adapt more swiftly and efficiently to changing market dynamics, thereby securing a competitive edge.
Streamlining Data Processing
AI automation's integration into predictive analytics has greatly streamlined data processing, enhancing efficiency and accuracy in data management systems. By leveraging AI, organizations can guarantee data integrity and bolster information security, two pillars essential for maintaining control over data landscapes.
- Automated data cleansing preserves data integrity by eliminating errors and inconsistencies.
- Advanced algorithms enhance information security by detecting and mitigating potential breaches.
- Real-time data processing allows for immediate insights, giving businesses a competitive edge.
- Scalable solutions adapt to growing data volumes, ensuring systems remain efficient.
- Reduction in manual data handling minimizes human error, further protecting data accuracy.
Through these advancements, AI automation empowers organizations with the tools needed to master their data, ensuring they remain agile and informed in a rapidly evolving digital landscape.
Enhancing Accuracy Levels
Incorporating AI automation into predictive analytics markedly boosts accuracy levels, enabling businesses to make more informed decisions based on precise data analysis. This advancement hinges on the utilization of sophisticated accuracy metrics and thorough error analysis, which AI algorithms are exceptionally adept at handling.
By systematically identifying and correcting inaccuracies in data sets, AI automation guarantees that predictive models are both reliable and robust. This meticulous approach to data verification not only minimizes the margin for error but also greatly enhances the overall quality of predictive insights.
Consequently, businesses can confidently leverage these refined analytics to streamline operations, bolster performance, and achieve a competitive edge, all while maintaining a high degree of control over their strategic decision-making processes.
Forecasting Future Trends
Harnessing the power of AI automation in predictive analytics enables businesses to anticipate future trends with unprecedented accuracy. This technological advancement provides a strategic edge by analyzing vast amounts of data, making historical comparisons, and conducting market sentiment analysis.
- Explore Past Data: AI explores past data, identifying patterns and outcomes to forecast future trends.
- Market Sentiment Analysis: Understanding public perception and its impact on market trends.
- Data-Driven Decisions: Empowering leaders to make informed choices based on predictive insights.
- Risk Management: Mitigating potential setbacks by foreseeing market fluctuations.
- Competitive Advantage: Staying ahead by adapting to predicted changes before they happen.
Embracing AI automation in predictive analytics positions businesses to navigate the future with confidence, leveraging data for a sustainable competitive edge.
Impact on Business Insights
Predictive analytics has greatly transformed the landscape of business insights, enabling companies to anticipate market trends and customer behavior with unprecedented accuracy. By harnessing the power of this advanced analytical approach, businesses are now equipped to perform detailed market segmentation, effectively categorizing their customer base into distinct groups with similar needs or behaviors. This capability not only enhances the precision of targeted marketing strategies but also optimizes product development to meet the specific demands of each segment.
Furthermore, understanding consumer behavior through predictive analytics allows companies to tailor their offerings and communication strategies in a way that resonates more deeply with their audience. This personalized approach not only increases customer satisfaction but also fosters loyalty, thereby driving long-term business success. Businesses that leverage predictive analytics gain a significant competitive advantage, as they are better positioned to respond proactively to emerging trends and changing consumer preferences.
Advanced Analytics Techniques
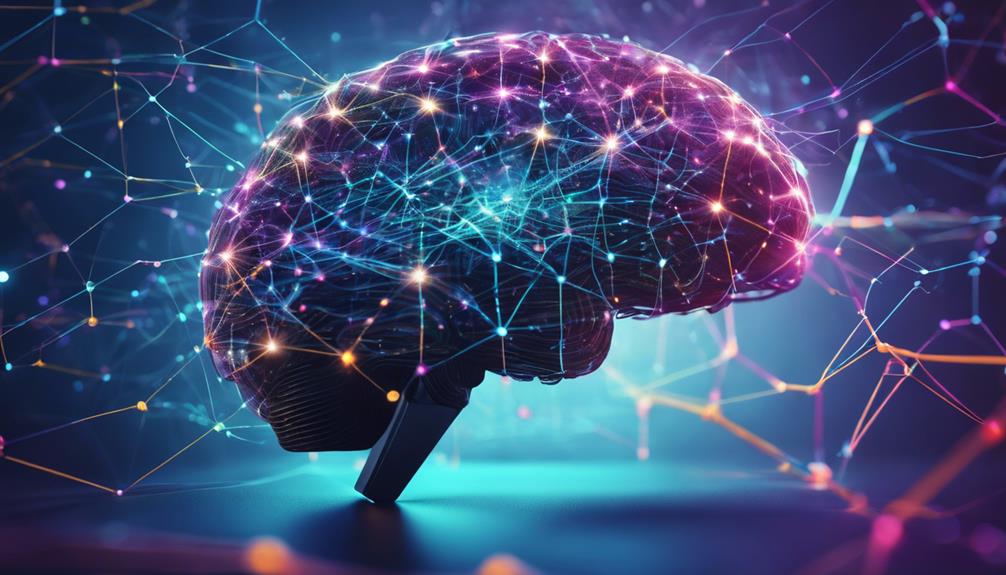
As we move into the domain of Advanced Analytics Techniques, it becomes imperative to understand the core components that are reshaping data management:
- Machine Learning Fundamentals
- Predictive Modeling Processes
- Data Mining Applications
These techniques not only enhance the accuracy of predictive analytics but also streamline the decision-making process, enabling businesses to anticipate future trends with greater precision.
Machine Learning Fundamentals
Machine Learning Fundamentals serve as the cornerstone of advanced analytics techniques, enabling data-driven decision-making through the automation of predictive modeling. These fundamentals are pivotal for organizations seeking control over their vast data landscapes, allowing them to harness the power of their information intelligently and efficiently.
Key considerations include:
- Understanding and mitigating algorithm biases for fairer outcomes
- Enhancing model interpretability to foster trust and adoption
- Leveraging large datasets to improve accuracy and reliability
- Employing cross-validation techniques to guarantee model robustness
- Prioritizing data quality and preprocessing to feed clean data into models
Predictive Modeling Processes
Exploring the domain of advanced analytics, the procedures of predictive modeling stand as a critical component, enabling businesses to forecast future trends and behaviors with remarkable accuracy. The process begins with algorithm selection, tailored to the specific nature of the dataset and the prediction objective. Following this, rigorous model evaluation guarantees the reliability of the predictions, ensuring that the model behaves as expected on unseen data. The table below outlines the key phases in the predictive modeling process:
Phase | Description | Key Considerations |
---|---|---|
Data Preparation | Cleansing and formatting data. | Accuracy, completeness. |
Algorithm Selection | Choosing the appropriate algorithm. | Suitability, efficiency. |
Model Training | Learning from the data. | Overfitting, underfitting. |
Model Validation | Evaluating model performance. | Precision, recall. |
This meticulous approach empowers businesses with the foresight needed to make informed decisions, driving their strategies forward with confidence.
Data Mining Applications
Data mining applications leverage advanced analytics techniques to unearth valuable insights from vast datasets, enhancing decision-making processes across various industries. These applications are pivotal in maneuvering the complexities of modern data landscapes, driving efficiency and innovation. However, they also raise pertinent issues such as privacy concerns and algorithmic bias that necessitate vigilant oversight.
- Customer Relationship Management: Tailoring services and products to meet customer needs more accurately.
- Fraud Detection: Identifying irregular patterns that may indicate fraudulent activity.
- Supply Chain Optimization: Enhancing efficiency and reducing costs through predictive analytics.
- Market Basket Analysis: Understanding customer purchasing patterns to optimize sales strategies.
- Healthcare Management: Improving patient care through predictive outcomes and personalized treatment plans.
Addressing privacy concerns and mitigating algorithmic bias are essential for harnessing the full potential of data mining applications responsibly.
Predictive Power in Action
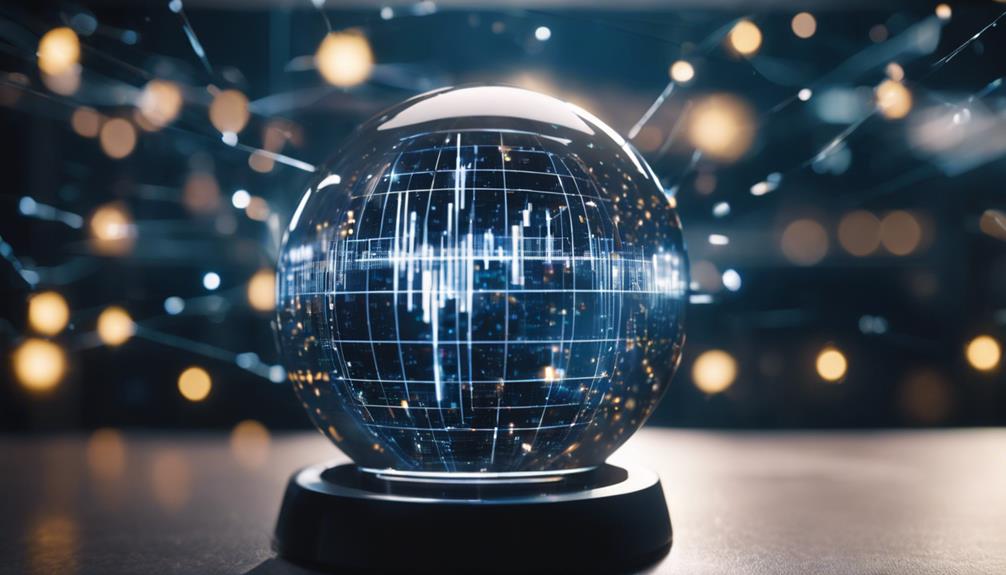
Harnessing the capabilities of predictive analytics, businesses are transforming decision-making processes with unprecedented accuracy and efficiency. Through the meticulous analysis of historical benchmarks and pattern identification, companies are now able to forecast future trends with a level of precision that was once deemed unattainable. This predictive power in action enables organizations to anticipate market changes, consumer behavior, and potential risks, thereby granting them a significant competitive edge.
The utilization of predictive analytics is not just about leveraging past data. It's about creating a strategic roadmap that guides decision-makers towards best outcomes. By analyzing historical trends and patterns, businesses can identify opportunities for growth and areas of improvement, ensuring that every decision is data-driven and strategically sound. This approach not only enhances operational efficiency but also boosts profitability and customer satisfaction.
In essence, the predictive power of analytics empowers businesses with the foresight to make informed decisions, minimize risks, and capitalize on emerging opportunities. It's a proof of how data, when analyzed and applied correctly, can serve as a powerful tool in shaping the future of business strategy and operations.
Future Trends in Data Management
Building on the foundation laid by predictive analytics, the landscape of data management is evolving rapidly to accommodate the next wave of technological advancements. As we navigate this transformation, several key trends are emerging that promise to redefine how we approach data management, ensuring that businesses retain control over their most valuable asset: their data.
- Data sovereignty is gaining prominence as organizations endeavor to navigate the complex web of international data regulations, ensuring data is stored and processed within legal boundaries.
- Regulatory compliance becomes more intricate, demanding sophisticated data management strategies to adapt to evolving legal frameworks.
- Automation in data governance will see advanced algorithms taking over routine data management tasks, reducing human error and freeing up resources for strategic initiatives.
- Real-time data processing is set to become the norm, enabling businesses to make quicker, more informed decisions.
- Enhanced data security measures will be essential in protecting against increasingly sophisticated cyber threats, ensuring data integrity and trust.
These trends represent a significant shift towards a more dynamic and responsive data management paradigm, where control, compliance, and security are paramount. As we look to the future, it's evident that mastering these trends will be essential for businesses aiming to leverage their data effectively in an ever-changing digital landscape.
Frequently Asked Questions
How Does Climate Change Affect Predictive Analytics in Data Management?
Climate change introduces variability, challenging data accuracy and algorithm resilience in predictive analytics. To maintain control, professionals must adapt models to account for this unpredictability, ensuring effective data management amidst evolving environmental conditions.
Can Predictive Analytics Predict Stock Market Trends Accurately?
Predictive analytics in forecasting stock market trends is akin to steering through a stormy sea; while algorithm accuracy improves, the inherent market volatility limits absolute predictability, offering investors a compass rather than a detailed map.
What Are the Ethical Considerations in Using Predictive Analytics?
Ethical considerations in predictive analytics encompass ensuring Bias Detection and promoting Algorithm Transparency. It's essential for stakeholders desiring control to mitigate unintended consequences and uphold fairness, thereby fostering a responsible application of predictive models in decision-making processes.
How Does Predictive Analytics Influence Consumer Privacy?
Predictive analytics walks a fine line, balancing knowledge power with consumer privacy. Incorporating data encryption and stringent privacy policies guarantees this technology respects user confidentiality, empowering individuals to maintain control over their personal information.
Are There Any Industries Where Predictive Analytics Is Not Effective?
Predictive analytics may not be as effective in creative industries where human intuition heavily influences outcomes. These sectors often value the unpredictability that comes with artistic creation, making data-driven predictions less impactful.
Conclusion
To conclude, predictive analytics propels the panorama of data management into unprecedented territories, enabling enterprises to embrace enhanced, efficient decision-making frameworks. By fortifying the foundation of business processes with predictive models, organizations achieve a superior stride in strategy and service.
The integration of advanced analytics techniques amplifies accuracy, augments insights, and accelerates the adoption of innovative practices. As the horizon of data management expands, the predictive prowess promises to be a pivotal piece in the puzzle of progress, shaping the future with foresight and finesse.